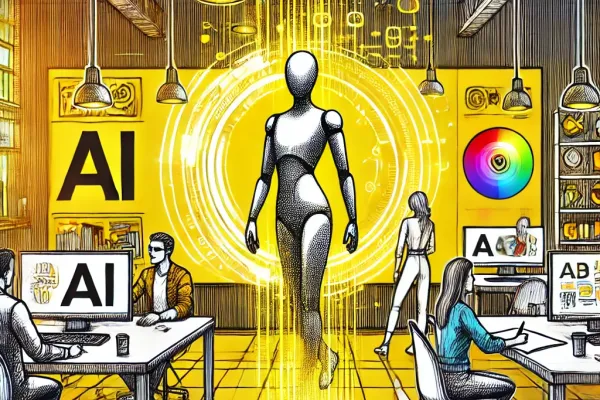
Details
The last 18 months have been chatbot this and LLM that, with much jawboning around what it may or may not do. But Machine Learning (ML) and Artificial Intelligence (AI) aren’t all chatbots, other opportunities can help the industry do what they do now, but better, faster, smarter.
Brand studios have been experimenting with AI tools on the content creation side but may have overlooked the potential, on the data and insights side. This is where there is a whole whitespace of opportunity to improve the work, drive performance and stimulate creativity and collaboration.
Studios can now build predictive models, take any new piece of content, and predict how well it will perform. This allows time for adjustments, and refinements to drive incremental performance. Adding this to the process helps leverage learnings from past work, reassure clients and create more creative freedom.
What will it look like in practice?
Imagine you are creating a bespoke piece of content for a brand, and you take the content and drop it in, to get a prediction of how well it will perform. The model uses your prior data and attributes about that content, to pattern match and predict how well your current piece will do.
This not only speeds up approval time, reduces back and forth but also reassures clients all through the process how you leverage data to drive performance for them. It creates better ROI for the studio’s efforts and the client’s budgets last longer.
This predictive model puts you in the driver's seat enabling you to make adjustments, ensure all content meets a performance standard, collaborate with your clients or provide feedback to your creative team empowering you to fine-tune it for maximum impact.
With predictive models, you can see how any proposed changes may impact performance. And make data-driven decisions. What a lift to any content strategy.
How brand studios can build a predictive model
This is a simplified explanation of the steps you can take to piece it together. It starts with building a historical model.
1) Historical data. Bring in all the performance data you have for the past two years–for every campaign.
Ideally, you want to go back as far as possible and collect all the campaign performance data you have. Preferable in a nice and tidy format, but do what you can, in as high a quality data as you can.
Examples of data sets could be:
- Attention
- Clicks
- Click rate
- Completion rate
- Band or survey data
- Conversion rates
- Average scroll
- View time.
Remember, gaps in data, or changes in metrics should be noted and split into different columns.
2) Analyze your past campaigns. Build a model which analyses your past content or campaigns. This must create a machine view of the content, or attributes which drive performance.
This is a bit more tricky, you need to create a tool, that analyses your campaign creatives be it video, content, or graphics. This tool helps turn it into data attributes, which can then be fed into a machine learning model. This enables the model to learn how these attributes impact the performance metrics and uncover patterns you might not have seen otherwise.
3) Fine-tune the model. Then fine fine-tune your predictive model based on this.
Now, you combine your historical data with machine learning algorithms to create a predictive model to forecast performance.
Typically you would take half the data you have collected, and you use that as your training set. Then the model will use the other half to make predictions, fine-tune itself and identify the attributes or variables most important to make that prediction.
What a predictive model enables
A predictive model enables you to analyse any new piece of work against past work.
- For every new content campaign you create, you’re leveraging all the intelligence from your prior campaigns. That is a vast and powerful repository. Potentially, millions or hundreds of millions of dollars in ad spend and insight.
- It proves to clients you are data-driven and opens up the field for creativity.
- You can bring the work in, predict how well it will perform and make adjustments to suit the creative work or the distribution.
This means you can return to older campaigns and find insights on making an idea or concept even better on a second attempt. Even bring them back to clients the next year.
Furthermore, you can circle back to clients with data-driven recommendations that support the creative work. The sales team love that, it helps them create new opportunities.
The use of a predictive model can fit several places in your current workflow:
- As part of pitching or responding to RFPs showing how you bring data-led insights into the process. They’re buying, you, your expertise and your strategic leverage of AI.
- In the creation process, testing concepts or creatives, to get feedback.
- As part of the sign-off, we want to see our work within these ranges.
- As an auditing and/or reporting process.
- Potentially examine competitive campaigns.